Hypertension, more commonly known as high blood pressure, affects an astonishing 1.28 billion adults worldwide. This condition arises when the force exerted by blood flow on the blood vessel walls becomes excessively high. Those with hypertension are at heightened risk for conditions like ischemic heart disease, stroke, and heart failure.
Often dubbed the “silent killer”, hypertension frequently goes unnoticed as many sufferers show no overt symptoms. Regular checks are the only definitive way to detect it. Although the exact cause often remains elusive, several risk factors, such as age, genetics, obesity, sedentary lifestyle, high salt intake, and excessive alcohol consumption, have been identified.
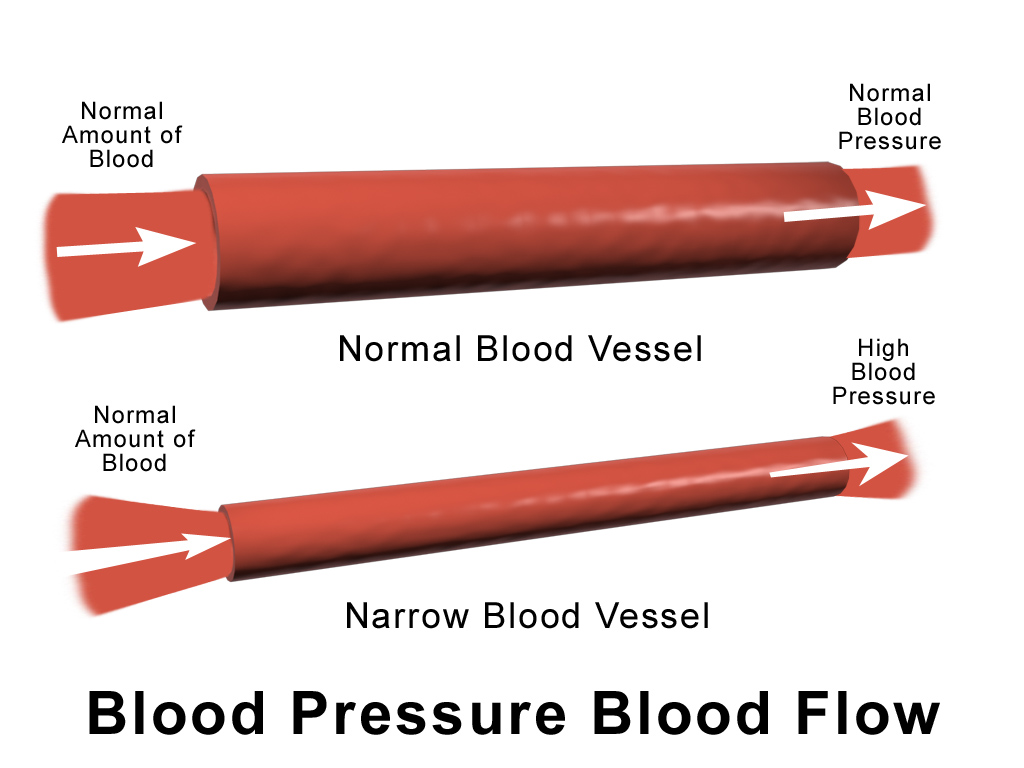
Traditionally, doctors advocate for lifestyle modifications as the first line of defense against hypertension. Then, doctors prescribe antihypertensive medications like angiotensin-converting enzyme (ACE) inhibitors, angiotensin II receptor blockers (ARBs), and calcium channel blockers if these changes prove insufficient.
It is believed that treatments for conditions like hypertension may need to be personalized to achieve higher efficacy. Yet, the sheer volume of patient data, coupled with the multitude of treatment options, makes practical personalization challenging.
Researchers have recently put forth a solution in a paper published in BMC Medical Informatics and Decision Making. They’ve leveraged machine learning to devise a model that suggests personalized hypertension treatments. This model takes into account factors like demographics, vital signs, past medical records, and clinical test outcomes.
This post may interest those with a higher polygenic risk score for high blood pressure. |
The Study
The researchers analyzed Electronic Health Records from Boston Medical Center (BMC), focusing on over 47,000 hypertensive patients. They collected pertinent demographic details and gauged prescription effectiveness by periodically reviewing blood pressure measurements.
Using this dataset, they engineered a machine learning algorithm aimed at predicting the optimal hypertension treatment for individual patients. For simplicity, they confined predictions to a single therapy.
Results
Personalized treatments using the model consistently outperformed. The best models achieved results that were a remarkable 70.30% better than the standard of care.
Predictions for those transitioning to a new therapy based on the model recommendations indicated even more substantial blood pressure reductions than if they had stuck with their current regimen.
Upon reviewing the treatments recommended by the model, the researchers found that BMC clinicians deemed a significant 87% of the model’s suggestions clinically sound. The recommendations that didn’t pass muster often pertained to combination treatments that clinicians found risky.
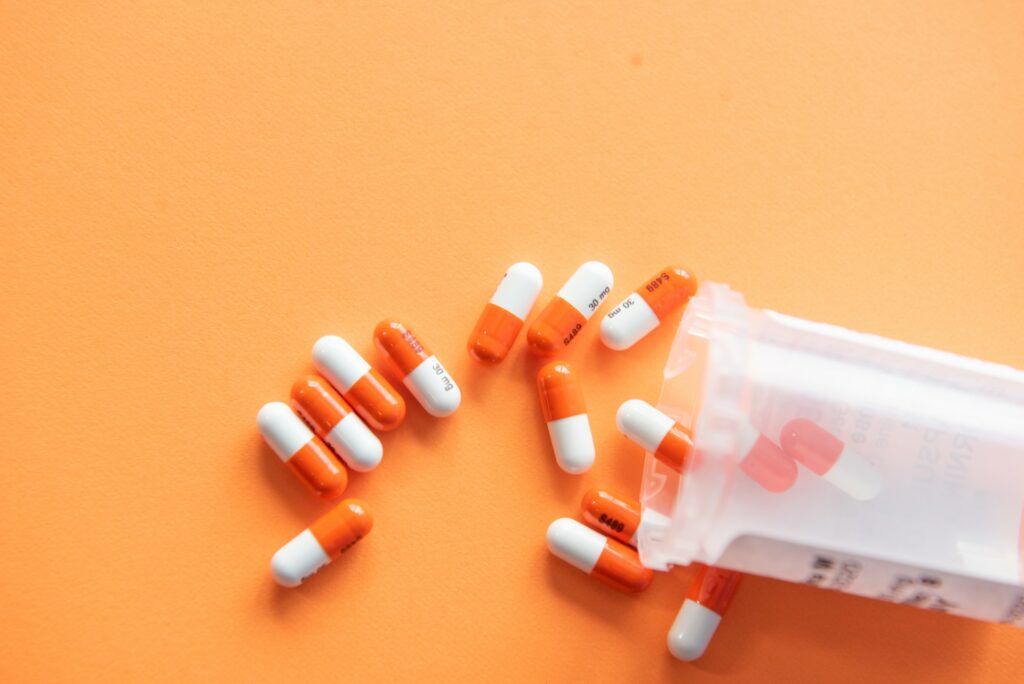
The model also showcased demographic trends. For instance, it often recommended ACEIs and beta-blockers for men, while it pointed women towards ARBs. Moreover, Black patients responded better to MRAs, whereas White patients fared better with beta-blockers. However, the authors caution that race, being intertwined with various environmental and socioeconomic factors, is likely representative of other unaccounted variables.
The study does have limitations. The study findings are predictive and haven’t been tested in real-world clinical scenarios. Additionally, factors outside their model, like drug allergies and past drug tolerances, need to be considered.
Summary
Harnessing the power of machine learning, this study presents a promising algorithm for personalized hypertension treatment predictions. Preliminary results indicate that these AI-driven prescriptions could outperform conventional medical recommendations. Such advancements in machine learning could bolster clinical decision-making, paving the way for better patient outcomes.
Citation
Hu, Y., Huerta, J., Cordella, N. et al. Personalized hypertension treatment recommendations by a data-driven model. BMC Med Inform Decis Mak 23, 44 (2023). https://doi.org/10.1186/s12911-023-02137-z